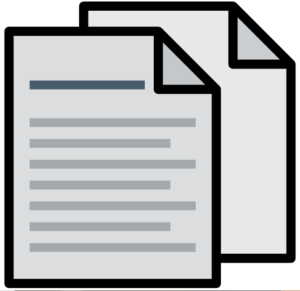
A General Framework of Multi-Agent Features Extraction Operators for Deformed Images Identification
Abstract: Texture extraction of images is a core step in image pattern recognition applications. Different operators are applied for image texture extraction in order to obtain a unique identification of an image texture based on physical characteristics of the images. These operators are categorized into local and global texture analysis descriptors. This categorization is based on the properties of the operators and their features extraction methodology. However, each operator of a type has its strength and weakness in handling the deformations of the images including rotation, shear, region of interest, noise and variance of intensity. Subsequently, there is no operator that can perfectly handle all the deformation problems. To this end, this paper proposes a general framework of a Collaborative Multi-Agent Feature Extraction (CMAFE). The CMAFE framework deploys a multi-agent system to perform dynamic collaborations between the feature extraction operators. The operators are formed in a multi-agent system in which each agent implements an operator. This collaboration between the agents is based on a divide and conquer strategy. This strategy enables the operators to cope with the various challenges of deformations that are affecting the accuracy of the identification. The framework is meant to improve the image identification accuracy and reduce the universal search space of local and global operators which in return reduce the computational cost and time.
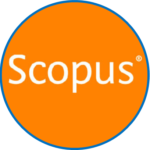