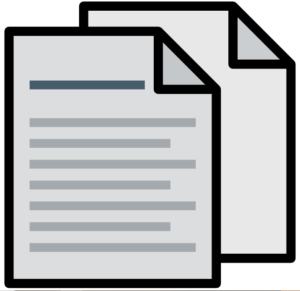
An Extra Tree Regression Model for Discharge Coefficient Prediction: Novel, Practical Applications in the Hydraulic Sector and Future Research Directions
Abstract Despite modern advances used to estimate the discharge coefficient (), it is still a major challenge for hydraulic engineers to accurately determine for side weirs. In this study, extra tree regression (ETR) was used to predict the of rectangular sharp-crested side weirs depending on hydraulic and geometrical parameters. The prediction capacity of the ETR model was validated with two predictive models, namely, extreme learning machine (ELM) and random forest (RF). The quantitative assessment revealed that the ETR model achieved the highest accuracy in the predictions compared to other applied models, and also, it exhibited excellent agreement between measured and predicted (correlation coefficient is 0.9603). Moreover, the ETR achieved 6.73% and 22.96% higher prediction accuracy in terms of root mean square error in comparison to ELM and RF, respectively. Furthermore, the performed sensitivity analysis shows that the geometrical parameter such as b/B has the most influence on . Overall, the proposed model (ETR) is found to be a suitable, practical, and qualified computer-aid technology for modeling that may contribute to enhance the basic knowledge of hydraulic considerations.
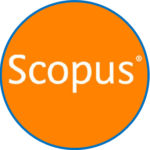