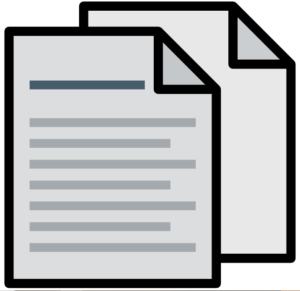
An intelligent evolutionary extreme gradient boosting algorithm development for modeling scour depths under submerged weir
Abstract This research presents a new hybridized evolutionary artificial intelligence (AI) model for modeling depth scouring under submerged weir (��). The proposed model is based on the hybridization of the Extreme Gradient Boosting (XGBoost) model and genetic algorithm (GA) optimizer. The GA is hybridized to solve the hyper-parameter problem of the XGBoost model and to recognize the influential input predictors of ��. The proposed XGBoost-GA model is developed based on the incorporation of fifteen physical parameters of submerged weir. The feasibility of the XGBoost-GA model is validated against several well-established AI models introduced in the literature in addition to a hybrid XGBoost-Grid model. Several statistical performance metrics is computed for the modeling evaluation in parallel with a graphical assessment. Based on the attained prediction results, the proposed model revealed an optimistic and superior predictability performance with a maximum coefficient of determination (�2 = 0.933) and a minimum root mean square error (RMSE = 0.014 m). In addition, the XGBoost-GA model
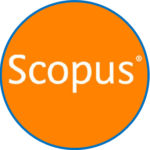